MySQL replication process enables you to reduce load MySQL and increase high availability. The benefit of MySQL replication also includes disaster recovery and reporting. In this tutorial, we will set up MySQL replication on 2 servers: one for the master database and another for the slave database. You can use virtual machines or VPS for this lab.
MySQL powers the most demanding Web, E-commerce, SaaS and Online Transaction Processing (OLTP) applications. It is a fully integrated transaction-safe, ACID compliant database with full commit, rollback, crash recovery and row level locking capabilities. MySQL delivers the ease of use, scalability, and performance to power Facebook, Google, Twitter, Uber, and Booking.com.
MySql replication is the process of creating the availability of the same database on some different remote location. The databases are always syn with each other. Depending on the configuration, you can replicate all databases, selected databases, or even selected tables within a database. Replication can be Mater-slave or Master-Master.
Sometimes you want to validate payload on a specific environment before submitting data to orches via API Proxy but you can’t apply any pre-existing policies. To achieve this, you must develop a custom policy for API Proxy.
If you forget password MySQL server on Windows and have tried all tutorials from mysql.com but it isn’t working. Don’t worry, I am too. It took me 3 hours to find the solution below to recover MySQL root password.
Docker Hub is a service provided by Docker for finding and sharing container images with your team. It allows us to pull and push docker images to and from Docker Hub. We can treat this as a GitHub, where we fetch and push our source code, but in the case of Docker Hub, we download or publish our container images.
To create a custom Docker image you will need a Dockerfile. This file describes all the steps that are required to create one image and would usually be contained within the root directory of the source .code repository for your application.
What is Docker?
Docker is an open-source project that automates the deployment of applications inside software containers. These application containers are similar to lightweight virtual machines, as they can be run in isolation to each other and the running host.
After discussed in detail the definitions of Apache Kafka, We’ll discuss about Kafka Stream and how to implement Kafka Stream in Spring Boot with Java.
What is Kafka stream?
Kafka Streams is a client library for building applications and microservices, where the input and output data are stored in Kafka clusters. It combines the simplicity of writing and deploying standard Java and Scala applications on the client side with the benefits of Kafka’s server-side cluster technology.
Why use Kafka Stream?
Core Kafka Streams concepts include: topology, time, keys, windows, KStreams, KTables, domain-specific language (DSL) operations, and SerDes.
Kafka Topology
A processor topology includes one or more graphs of stream processors (nodes) connected by streams (edges) to perform stream processing. In order to transform data, a processor receives an input record, applies an operation, and produces output records.
A source processor receives records only from Kafka topics, not from other processors. A sink processor sends records to Kafka topics, and not to other processors.
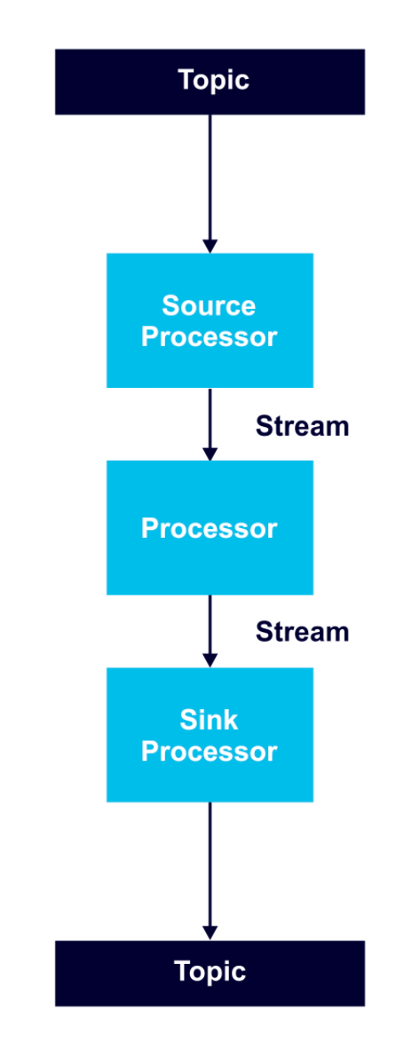
Processor nodes can run in parallel, and it is possible to run multiple multi-threaded instances of Kafka Streams applications. However, it is necessary to have enough topic partitions for the running stream tasks, since Kafka leverages partitions for scalability.
Stream tasks serve as the basic unit of parallelism, with each consuming from one Kafka partition per topic and processing records through a processor graph of processor nodes.
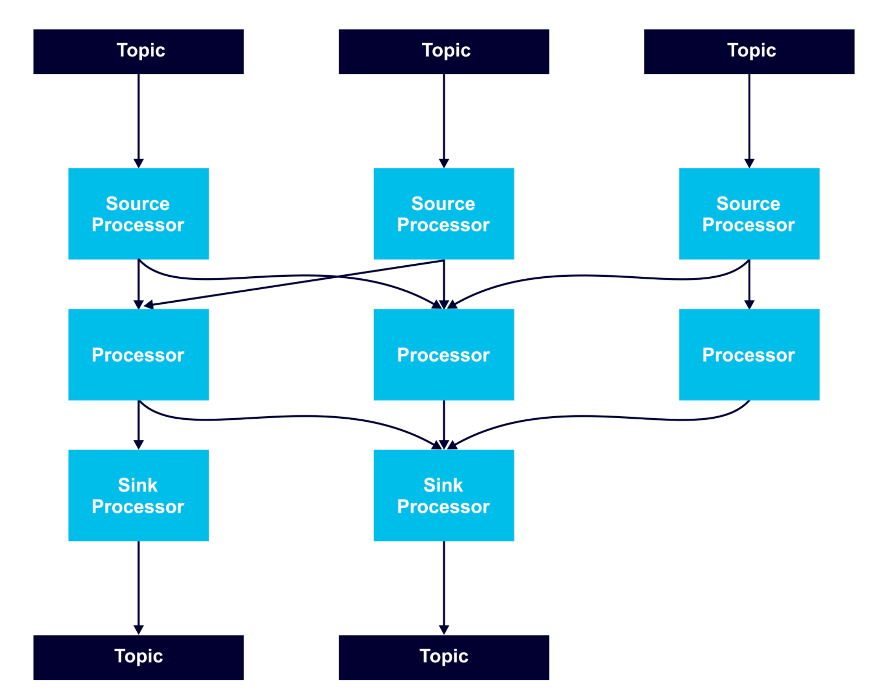
To keep partitioning predictable and all stream operations available, it is a best practice to use a record key in records that are to be processed as streams.
Time
Time is a critical concept in Kafka Streams. Streams operations that are windowing-based depend on time boundaries. Event time is the point in time when an event is generated. Processing time is the point in time when the stream processing application consumes a record. Ingestion time is the point when an event or record is stored in a topic. Kafka records include embedded time stamps and configurable time semantics.
Domain-Specific Language (DSL) built-in abstractions
The DSL is a higher level of abstraction. The methods of the DSL let you think about how you want to transform the data rather than explicitly creating a processor Topology. The Topology is built for you with the StreamsBuilder#build() method.
The Streams DSL offers streams and tables abstractions, including KStream, KTable, GlobalKTable, KGroupedStream, and KGroupedTable.
Developers can leverage the DSL as a declarative functional programming style to easily introduce stateless transformations such as map and filter operations, or stateful transformations such as aggregations, joins, and windowing.
KTables
KTable
is an abstraction of a changelog stream from a primary-keyed table. Each record in this changelog stream is an update on the primary-keyed table with the record key as the primary key.
A KTable
is either defined from a single Kafka topic
that is consumed message by message or the result of a KTable
transformation. An aggregation of a KStream
also yields a KTable
.
A KTable
can be transformed record by record, joined with another KTable
or KStream
, or can be re-partitioned and aggregated into a new KTable
.
Some KTable
s have an internal state (a ReadOnlyKeyValueStore
) and are therefore queryable via the interactive queries API.
For example
final KTable table = ...
...
final KafkaStreams streams = ...;
streams.start()
...
final String queryableStoreName = table.queryableStoreName(); // returns null if KTable is not queryable
ReadOnlyKeyValueStore view = streams.store(queryableStoreName, QueryableStoreTypes.keyValueStore());
view.get(key);
DSL Operations
The following table offers a quick-and-easy reference for understanding all DSL operations and their input and output mappings, in order to create streaming applications with complex topologies:
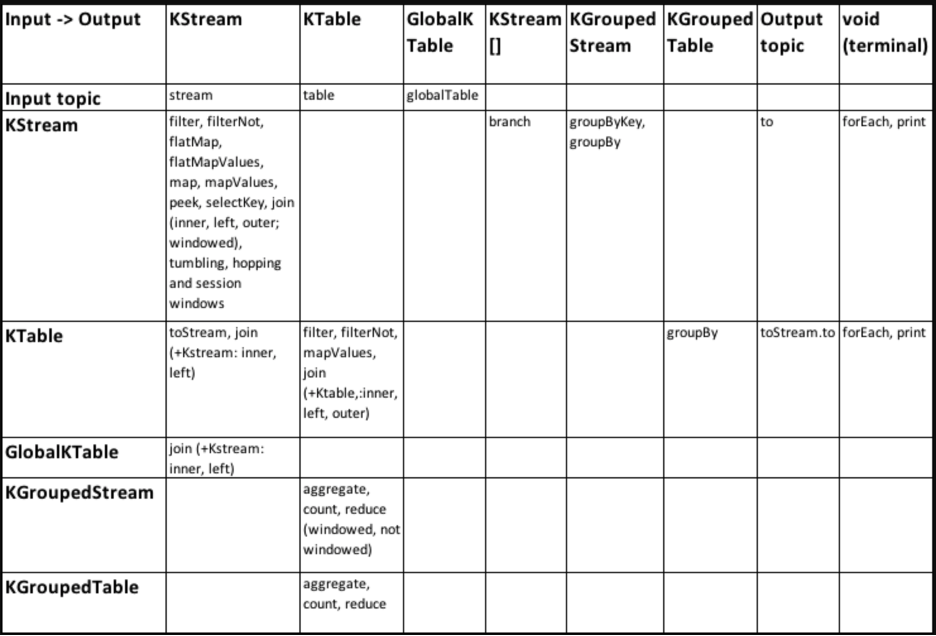
SerDes
Kafka Streams applications need to provide SerDes, or a serializer/deserializer, when data is read or written to a Kafka topic or state store. This enables record keys and values to materialize data as needed. You can also provide SerDes either by setting default SerDes in a StreamsConfig instance, or specifying explicit SerDes when calling API methods.
The following diagram displays SerDes along with other data conversion paths:
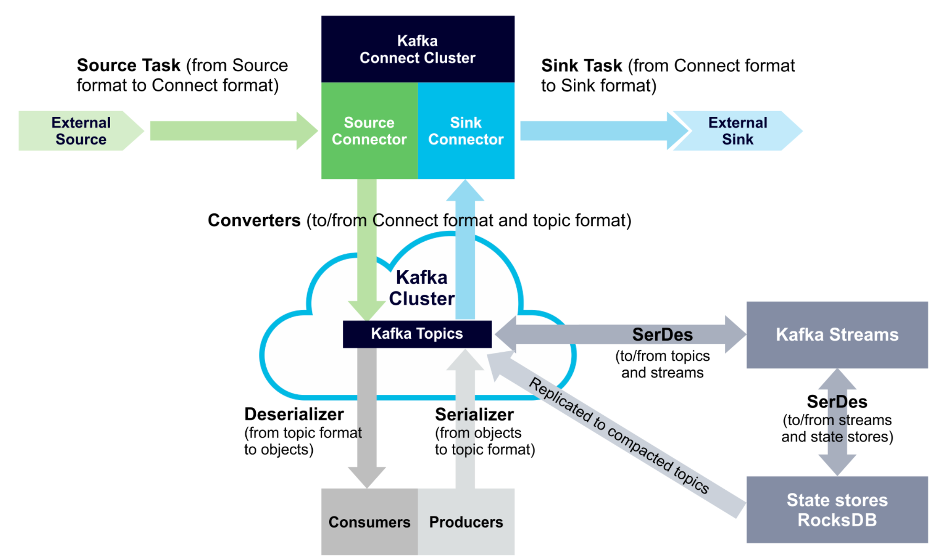
Demo Kafka stream
Below is a demo Kafka stream: build word count application.
package com.learncode24h.wordcount;
import org.apache.kafka.clients.consumer.ConsumerConfig;
import org.apache.kafka.common.serialization.Serdes;
import org.apache.kafka.streams.KafkaStreams;
import org.apache.kafka.streams.StreamsBuilder;
import org.apache.kafka.streams.StreamsConfig;
import org.apache.kafka.streams.kstream.KStream;
import org.apache.kafka.streams.kstream.KTable;
import org.apache.kafka.streams.kstream.Produced;
import java.io.FileInputStream;
import java.io.IOException;
import java.util.Arrays;
import java.util.Locale;
import java.util.Properties;
import java.util.concurrent.CountDownLatch;
/**
* Demonstrates, using the high-level KStream DSL, how to implement the WordCount program
* that computes a simple word occurrence histogram from an input text.
* <p>
* In this example, the input stream reads from a topic named "streams-plaintext-input", where the values of messages
* represent lines of text; and the histogram output is written to topic "streams-wordcount-output" where each record
* is an updated count of a single word.
* <p>
* Before running this example you must create the input topic and the output topic (e.g. via
* {@code bin/kafka-topics.sh --create ...}), and write some data to the input topic (e.g. via
* {@code bin/kafka-console-producer.sh}). Otherwise you won't see any data arriving in the output topic.
*/
public final class WordCountDemo {
public static final String INPUT_TOPIC = "streams-plaintext-input";
public static final String OUTPUT_TOPIC = "streams-wordcount-output";
static Properties getStreamsConfig(final String[] args) throws IOException {
final Properties props = new Properties();
if (args != null && args.length > 0) {
try (final FileInputStream fis = new FileInputStream(args[0])) {
props.load(fis);
}
if (args.length > 1) {
System.out.println("Warning: Some command line arguments were ignored. This demo only accepts an optional configuration file.");
}
}
props.putIfAbsent(StreamsConfig.APPLICATION_ID_CONFIG, "streams-wordcount");
props.putIfAbsent(StreamsConfig.BOOTSTRAP_SERVERS_CONFIG, "localhost:9092");
props.putIfAbsent(StreamsConfig.CACHE_MAX_BYTES_BUFFERING_CONFIG, 0);
props.putIfAbsent(StreamsConfig.DEFAULT_KEY_SERDE_CLASS_CONFIG, Serdes.String().getClass().getName());
props.putIfAbsent(StreamsConfig.DEFAULT_VALUE_SERDE_CLASS_CONFIG, Serdes.String().getClass().getName());
props.putIfAbsent(ConsumerConfig.AUTO_OFFSET_RESET_CONFIG, "earliest");
return props;
}
static void createWordCountStream(final StreamsBuilder builder) {
final KStream<String, String> source = builder.stream(INPUT_TOPIC);
final KTable<String, Long> counts = source
.flatMapValues(value -> Arrays.asList(value.toLowerCase(Locale.getDefault()).split(" ")))
.groupBy((key, value) -> value)
.count();
// need to override value serde to Long type
counts.toStream().to(OUTPUT_TOPIC, Produced.with(Serdes.String(), Serdes.Long()));
}
public static void main(final String[] args) throws IOException {
final Properties props = getStreamsConfig(args);
final StreamsBuilder builder = new StreamsBuilder();
createWordCountStream(builder);
final KafkaStreams streams = new KafkaStreams(builder.build(), props);
final CountDownLatch latch = new CountDownLatch(1);
// attach shutdown handler to catch control-c
Runtime.getRuntime().addShutdownHook(new Thread("streams-wordcount-shutdown-hook") {
@Override
public void run() {
streams.close();
latch.countDown();
}
});
try {
streams.start();
latch.await();
} catch (final Throwable e) {
System.exit(1);
}
System.exit(0);
}
}
Deploy
To deploy this application we need to create input topic and output topic
# Create the input topic
./bin/kafka-topics --create \
--bootstrap-server localhost:9092 \
--replication-factor 1 \
--partitions 1 \
--topic streams-plaintext-input
# Create the output topic
./bin/kafka-topics --create \
--bootstrap-server localhost:9092 \
--replication-factor 1 \
--partitions 1 \
--topic streams-wordcount-output
Create input_file.txt for producer with the following content
welcome to learncode24h.com we are learning kafka streams thanks for reading kafka streams
Lastly, we send this input data to the input topic:
cat /tmp/file-input.txt | ./bin/kafka-console-producer --broker-list localhost:9092 --topic streams-plaintext-input
We can now inspect the output of the WordCount demo application by reading from its output topic streams-wordcount-output
./bin/kafka-console-consumer --bootstrap-server localhost:9092 \
--topic streams-wordcount-output \
--from-beginning \
--formatter kafka.tools.DefaultMessageFormatter \
--property print.key=true \
--property key.deserializer=org.apache.kafka.common.serialization.StringDeserializer \
--property value.deserializer=org.apache.kafka.common.serialization.LongDeserializer
In Apache Kafka , producer and consumer performance are very similar in that both are concerned with throughput.
Consumer Lag
For producers, we care mostly about how fast the producer is sending messages to the broker. Obviously, the higher the throughput, the better.
For consumers, we are also concerned with performance, or how fast we can read messages from a broker. But there is another way to measure consumer performance: consumer lag. The difference between how fast the producers place records on the broker and when consumers read those messages is called consumer lag.
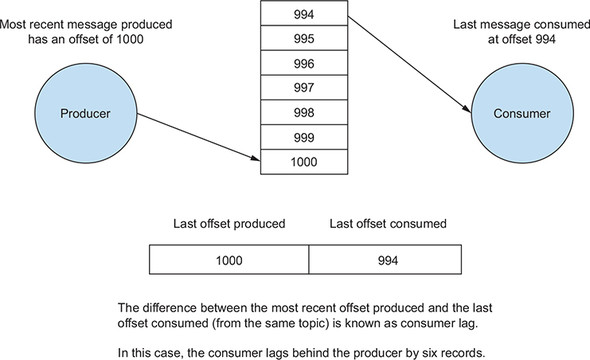
Monitor Consumer Lag
To check for consumer lag, Kafka provides a convenient command-line tool, kafka-consumer-groups.sh, found in the <kafka-install-dir>/bin directory. The script has a few options, but here we focus on the list and describe options. These two options will give you the information you need about consumer group performance.
The kafka-consumer-groups
tool can be used to list all consumer groups, describe a consumer group, delete consumer group info, or reset consumer group offsets.
Use the following command to describe offsets committed to Kafka:
kafka-consumer-groups.bat --bootstrap-server 127.0.0.1:9092 --describe --group first_app
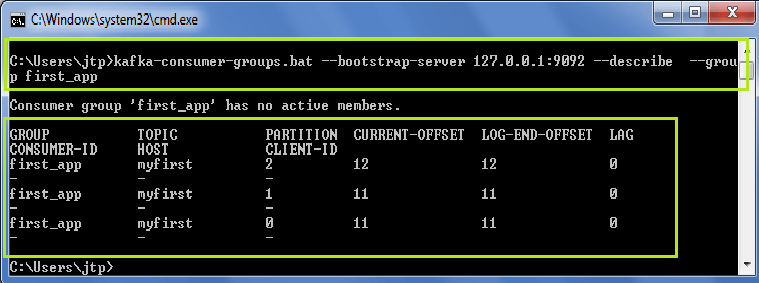
Lag value is 0 -indicates that the consumer has read all the data: 12 messages sent – 12 message read = 0 lag (or records behind), similar for other consumers.
Kafka ACKS and ISR (In-Sync Replicas)
Those two configs are acks
and min.insync.replicas
have a big impact on performance of Kafka. So in this tutorial we’ll dig deep about them.
Replication
For each partition, there exists one leader broker and follower brokers.
The config which controls how many such brokers (1 + N) exist is replication.factor
. That is the total amount of times the data inside a single partition is replicated across the cluster.
The default and typical recommendation is three.
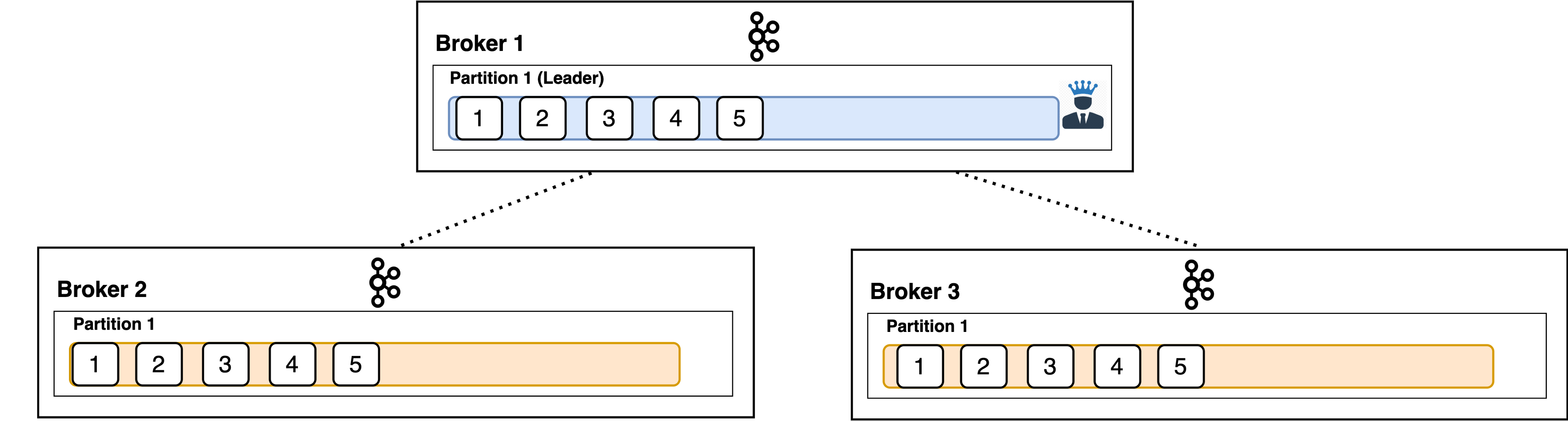
Producer clients only write to the leader broker’s followers asynchronously to replicate the data.
In-sync replicas
An in-sync replica (ISR) is a broker that has the latest data for a given partition. In other words, In-Sync Replicas are the replicated partitions that are in sync with their leader, i.e. those followers that have the same messages (or in sync) as the leader. It is not mandatory to have ISR equal to the number of replicas.
A leader is always an in-sync replica. A follower is an in-sync replica only if it has fully caught up to the partition it is following. In other words, it can not be behind on the latest records for a given partition.
If a follower broker falls, after 10 seconds it will be removed from the ISR. Likewise, if a follower slows down, perhaps a network-related issue or constrained server resources, then as soon as it has been lagging behind the leader for more than 10 seconds it is removed from the ISR.
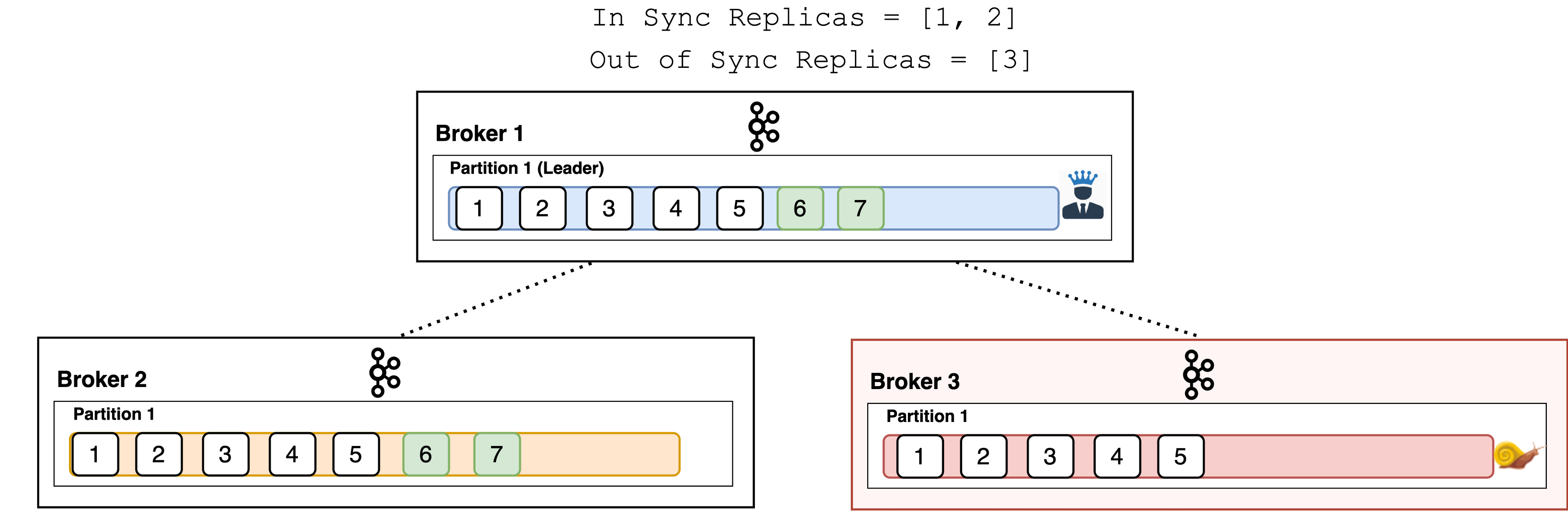
Instead of checking after 10 seconds you can configure this time period at: replica.lag.time.max.ms
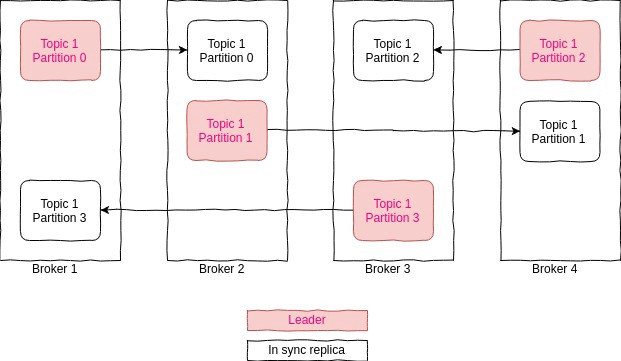
when we say a topic has a replication factor of 2 that means we will be having two copies of each of its partitions. Kafka considers that a record is committed when all replicas in the In-Sync Replica set (ISR) have confirmed that they have written the record to disk.
Acknowledgements (ACKS)
The acks
setting is a client (producer) configuration. It denotes the number of brokers that must receive the record before we consider the write as successful. It supports three values are: 0
, 1
, and all
.
acks=0
With a value of 0
, the producer won’t even wait for a response from the broker. It immediately considers the write successful the moment the record is sent out.
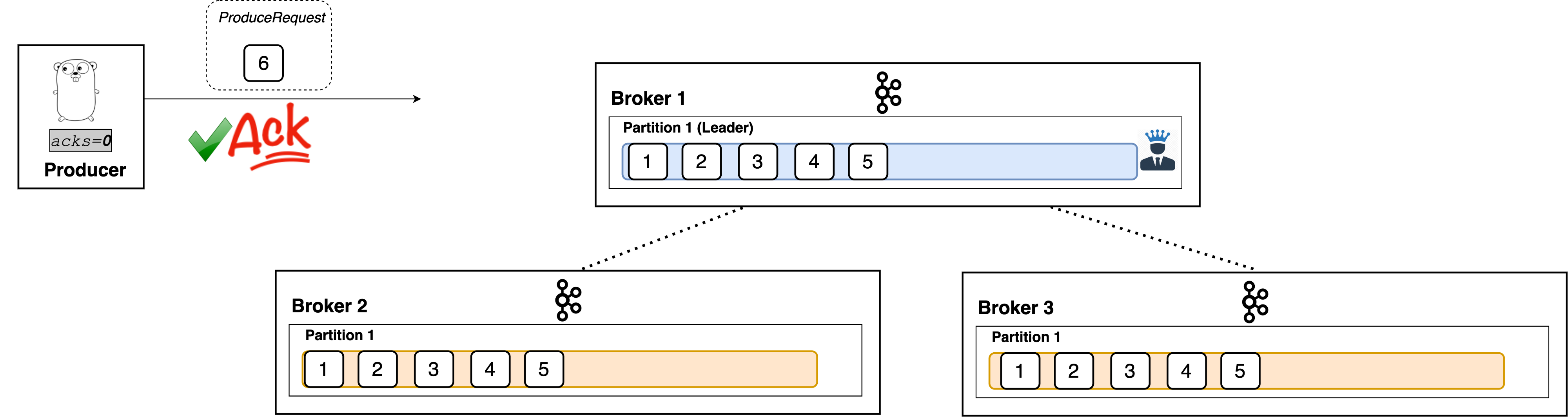
acks=1
With a setting of 1
, the producer will consider the write successful when the leader receives the record. The leader broker will know to immediately respond the moment it receives the record and not wait any longer.
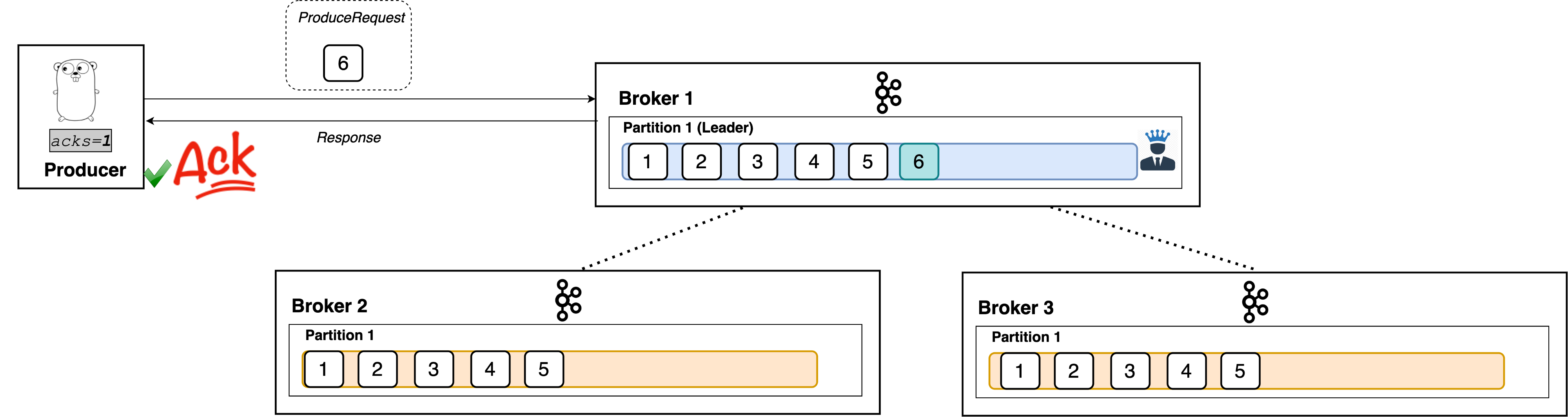
acks=all
When set to all
, the producer will consider the write successful when all of the in-sync replicas receive the record. This is achieved by the leader broker being smart as to when it responds to the request it will send back a response once all the in-sync replicas receive the record themselves.
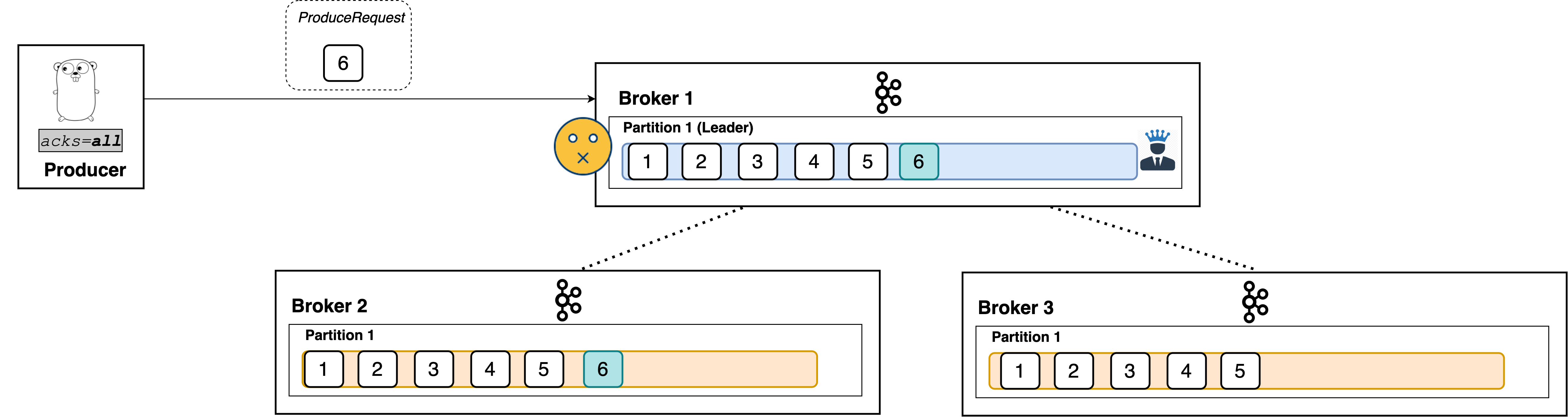
Like I said, the leader broker knows when to respond to a producer that uses acks=all
.
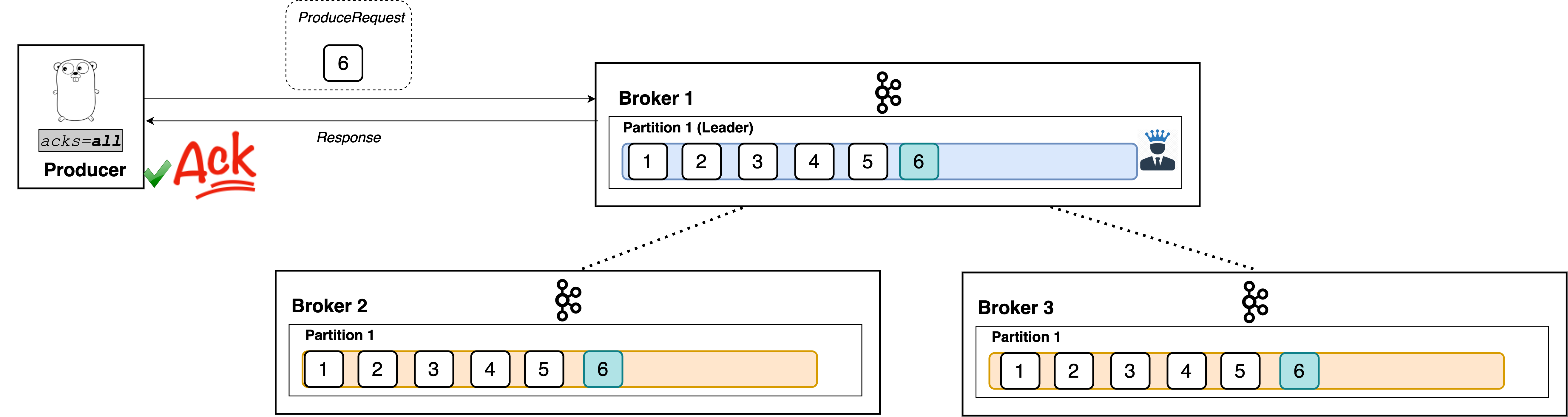
Minimum In-Sync Replica
min.insync.replicas
is a config on the broker that denotes the minimum number of in-sync replicas required to exist for a broker to allow acks=all
requests. That is, all requests with acks=all
won’t be processed and receive an error response if the number of in-sync replicas is below the configured minimum amount. It acts as a sort of gatekeeper to ensure scenarios like the one described above can not happen.
If this minimum cannot be met, then the producer will raise an exception (either NotEnoughReplicas or NotEnoughReplicasAfterAppend).
As shown, min.insync.replicas=X
allows acks=all
requests to continue to work when at least x replicas of the partition are in sync. Here, we saw an example with two replicas.
But if we go below that value of in-sync replicas, the producer will start receiving exceptions.
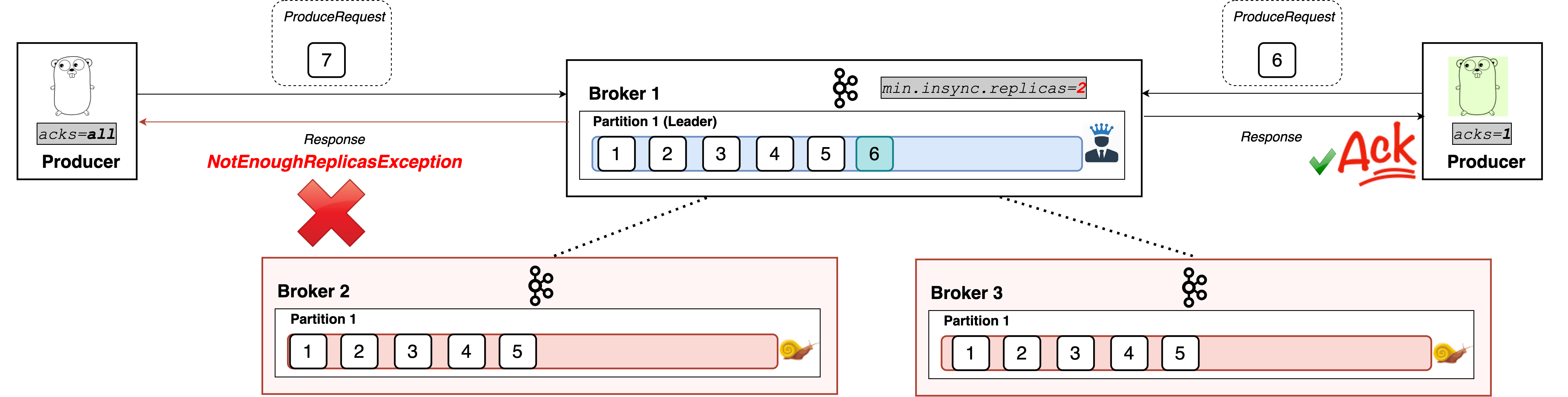
As you can see, producers with acks=all
can’t write to the partition successfully during such a situation. Note, however, that producers with acks=0
or acks=1
continue to work just fine.
Caveat
A common misconception is that min.insync.replicas
denotes how many replicas need to receive the record in order for the leader to respond to the producer. That is not true: the config is the minimum number of in-sync replicas required to exist in order for the request to be processed.
That is, if there are three in-sync replicas and min.insync.replicas=2
, the leader will respond only when all three replicas have the record.
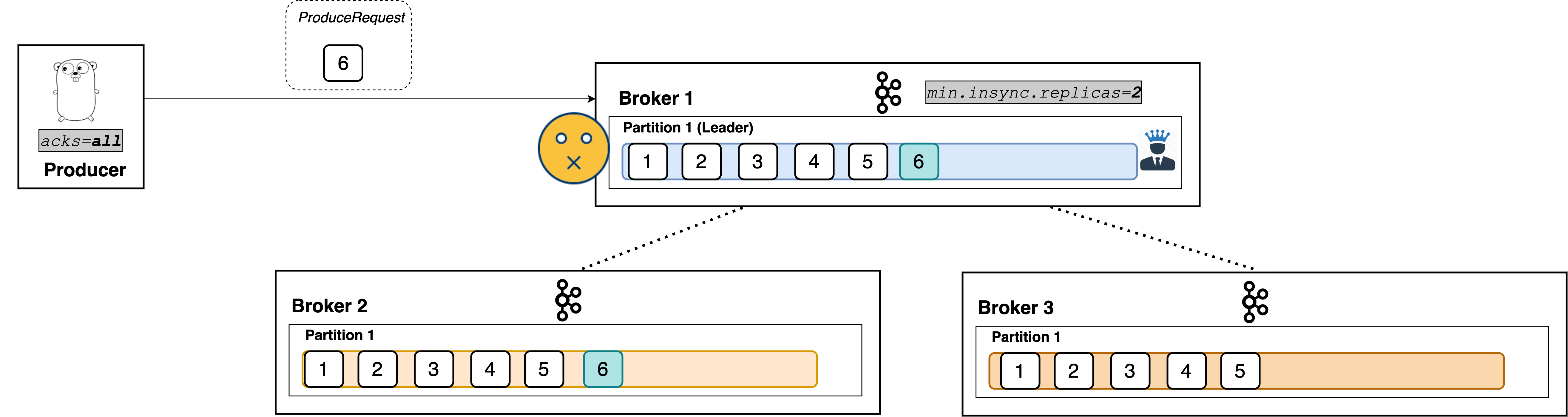
Summary
To recap, acks
and min.insync.replicas
settings are what let you configure the preferred durability requirements for writes in your Kafka cluster.
acks=0
the write is considered successful the moment the request is sent out. No need to wait for a response.acks=1
the leader must receive the record and respond before the write is considered successful.acks=all
all online in sync replicas must receive the write. If there are less thanmin.insync.replicas
online, then the write won’t be processed.